AI Law for Innovators: Navigating the "Law of Guardrails" and "Ethical Bias"
AI Law for Innovators: Navigating the "Law of Guardrails" and "Ethical Bias"
By Ira P. Rothken
Summary of Key Points:
• Explore the emerging "Law of Guardrails" - a crucial legal paradigm for ensuring ethical, compliant AI development in the era of powerful large language models (LLMs).
• Discover how "Macro and Micro Guardrails", implemented through code by lawyers and engineers, act as compliance firewalls for AI systems, optimizing relevance and preventing harmful outputs.
• Understand the nuances of "ethical bias" and its necessary role in aligning AI with diverse organizational goals, from brand protection to religious values to legal advocacy.
• Navigate the balance between neutral AI outputs and the need for customizable guardrails that respect global diversity in laws, norms, and definitions of acceptable content.
• Prepare for a future where collaborative efforts among legal, policy, and technology experts will shape responsible AI innovation through the intricate "Law of Guardrails".
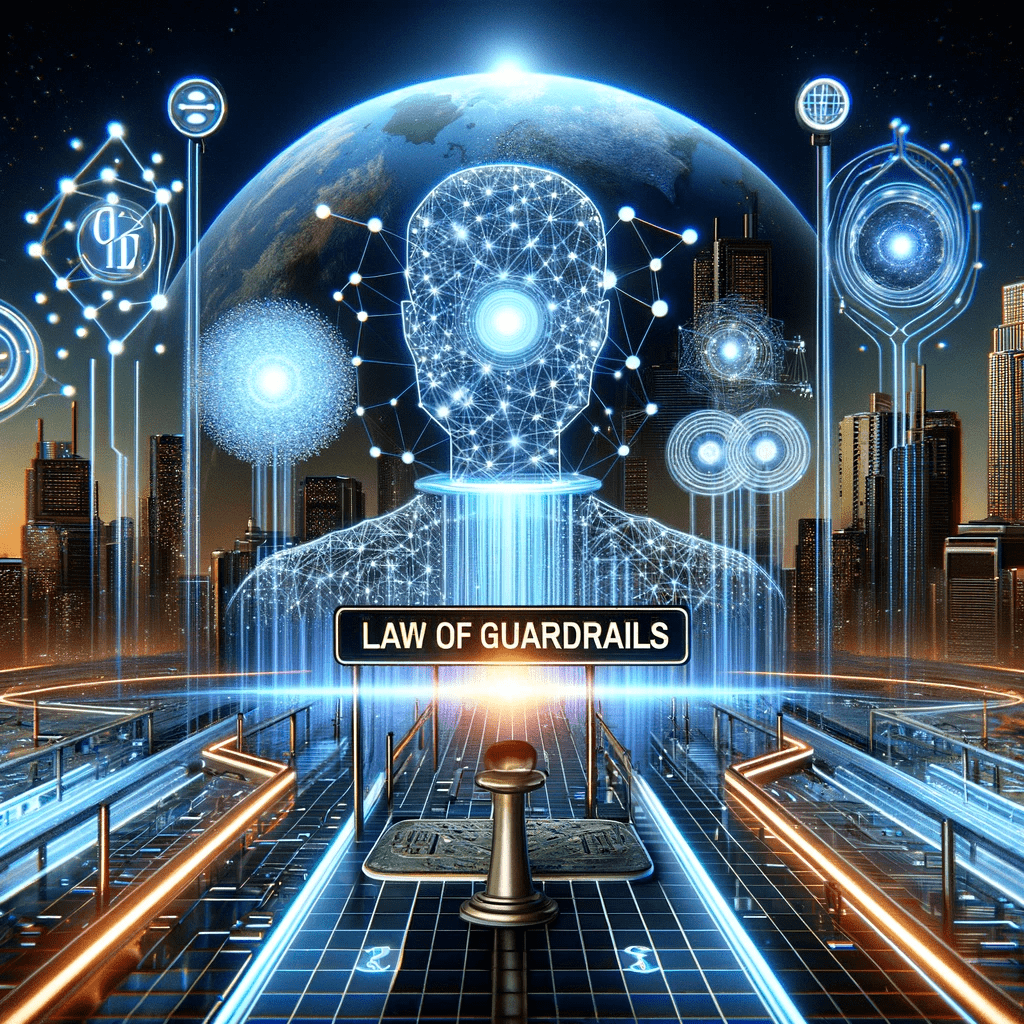
Introduction
The advent of artificial intelligence (AI) and large language models (LLMs) such as ChatGPT, Claude, and Gemini has ushered in a new era of technological innovation, transforming industries and redefining the boundaries of what is possible. As these AI systems become increasingly sophisticated and resource-intensive, the majority of AI LLM applications by organizations have begun to rely on API calls to these industry leaders. This shift has highlighted the importance of implementing "Micro-Guardrails" - a set of rules and protocols designed to optimize relevance and ensure compliance with Environmental, Social, and Governance (ESG) policies, regulations, and legal requirements. The concept of "Retrieval-Augmented Generation" (RAG) has emerged as a key tool in assisting with prompt inputs and LLM outputs, further emphasizing the need for multi disciplinary design between lawyers, compliance officers, and engineers to create effective guardrails. As a result, the future of AI law is increasingly centered around the "Law of Guardrails" (LOG), a new legal paradigm that aims to ensure the ethical and compliant development of AI systems. This article explores the importance of “Macro and Micro Guardrails” in AI, the concept of “ethical bias,” and how they can be effectively implemented by lawyers, engineers, and compliance officers to optimize responsible use of AI LLMs.
The Essence of Guardrails in AI Development
Guardrails in AI are essential across various domains to ensure that AI systems operate within ethical, religious, and legal boundaries. These guardrails can be implemented at both the macro level, within the AI LLM itself, and at the micro level, through an organization's API calls, prompts, and outputs. These guardrails are designed to prevent AI applications from producing unintended or harmful outcomes, such as irrelevant outputs, privacy violations, or intellectual property infringements. For instance, in healthcare, AI systems used for diagnostics must have guardrails to protect patient data privacy and ensure the accuracy of medical diagnoses. Similarly, in finance, AI algorithms for credit scoring or fraud detection must incorporate guardrails to prevent discriminatory practices and protect sensitive financial information.
Guardrails in Practice: Implementing Compliance Firewalls for LLM API Calls and Outputs
To ensure that AI systems powered by large language models (LLMs) operate within ethical and legal boundaries, companies must implement guardrails that act like a compliance firewall. These guardrails are designed to modify the prompts to LLM API calls and outputs to optimize relevance to the use case and prevent the generation of harmful content, such as toxic words or trade secret violations.
Retrieval-Augmented Generation (RAG), commonly used to query credible databases of information, plays a crucial role in enhancing the effectiveness of guardrails in LLMs. By incorporating RAG, guardrails can be designed to ensure that the AI LLM generates outputs that are not only relevant to the given context but also grounded in factual information, thereby reducing the likelihood of hallucinations or inaccurate outputs.
1. API Calls and Prompt Engineering: Companies can design prompts carefully to guide the LLM towards generating relevant and appropriate responses. For instance, a legal research tool powered by an LLM might parse user prompts to discern keywords for a RAG call or query to a caselaw database and generate an internal prompt with more specific legal terms and context to the LLM API so that the generated output is more likely helpful to the user and legal issue at hand.
2. Output Filtering: Guardrails can include mechanisms to filter the outputs of LLMs, removing or flagging any content that contains toxic words, hate speech, off-topic, company policy or ESG non-compliance, or other inappropriate language. This can be achieved through keyword filtering, sentiment analysis, or more sophisticated natural language processing techniques. For example, RAG can be used to parse the potential LLM output to look up legal citations in text to discern if they actually exist in a credible database and if not then take actions to regenerate the output or suppress the hallucination or erroneous content.
3. Data Anonymization: To prevent the inadvertent disclosure of sensitive information, guardrails can anonymize data used in prompts or contained in outputs. This is particularly important in industries dealing with confidential information, such as healthcare or finance.
4. Access Controls: Implementing access controls as part of the guardrails ensures that only authorized users can make API calls to the LLM and view the outputs. This helps prevent unauthorized access to sensitive data and reduces the risk of trade secret violations.
5. Audit Trails: Maintaining audit trails of all interactions with the LLM, including prompts and outputs, is essential for compliance. This allows companies to review and analyze the use of the AI system, identify any potential issues, and demonstrate compliance with regulations and policies.
6. Regular Updates and Monitoring: Guardrails should be regularly updated and monitored to adapt to evolving legal, ethical, and technological standards. This includes updating keyword filters, refining prompt engineering and RAG techniques, and monitoring for emerging risks.
AI Law is Quickly Becoming the Law of Guardrails
Savvy tech lawyers are needed to play a crucial role in the evolution of AI especially when it comes to AI LLMs by using software code, and soon “no code” systems, to implement compliance by code design, this brings in the important AI era of the “Law of Guardrails.”
Lawyers will need to help develop custom guardrails using legal-tech and reg-tech for each client’s use case. For example:
1. Data Privacy and Security: Guardrails must protect the privacy and security of data processed by AI systems, complying with regulations such as GDPR and CCPA.
2. Intellectual Property Rights: AI systems must respect intellectual property rights, with guardrails mitigating unauthorized use or reproduction of copyrighted materials by users.
3. Transparency and Accountability: Guardrails should promote transparency and accountability in AI decision-making processes.
Lawyers Must Balance Client Needs at Different AI Use Case Levels
The Law of Guardrails is complex and involves a balance for each use case. The Macro-Guardrails suited for a general consumer AI LLM chatbot, like Chatgpt, that deals with the widest possible range of users from students to professionals is usually different than a specific and customized chatbot meant for a specific organization, or Micro-Guardrails.
It is crucial to strike a balance between avoiding the suppression of free speech and providing the flexibility for businesses to implement localized and customized guardrails that meets their compliance needs. The AI LLM platforms for their API deployment, as opposed to their consumer facing general purpose chatbots, should aim to offer Macro Guardrails for neutral, unbiased outputs while allowing downstream organizations that make API calls to tailor Micro-Guardrails based on their organization's specific and evolving needs. Organizations globally for example in the US, China, Poland, Africa, the Middle East, and the Vatican ought to get to choose their own Micro-Guardrails based on their goals, compliance policies, and cultural norms.
1. Neutral Output Policy: AI LLM platforms should strive to provide outputs that are neutral and free from inherent biases. This means that the major platforms should avoid using a heavy hand in imposing their own definitions of bias or other subjective standards but rather provide a base output that downstream organizations can then modify according to their requirements.
2. Customizable Guardrails: Businesses around the world operate in diverse legal and cultural landscapes. Lawyers working with engineers and no code tools will allow companies to customize guardrails according to their localized needs. For example, a company in a country with strict data privacy laws might prioritize guardrails around data anonymization, or an organization that is religious in nature might be more sensitive to offensive or disparaging outputs, while a company in a region with different free speech norms might focus on content moderation differently.
3. Transparency and Control: To facilitate customization, major LLM platforms should provide transparency about how their models implement Macro-Guardrails and generate outputs and support Micro-Guardrails control mechanisms to organizations through development of industry standards and tools. This can include detailed documentation, APIs for setting Micro-Guardrail parameters, and options for API users and organizations to train the models with their own data sets.
4. Respecting Local Laws and Norms: It is essential for major LLM platforms to respect the legal and cultural norms of the regions in which they operate. This means providing the flexibility for organizations and their counsel to adapt the AI outputs to comply with local regulations and societal standards, including varying definitions of bias and appropriate content.
5. Collaboration and Feedback: Major LLM platforms should actively collaborate with businesses and regulatory bodies to understand their needs and incorporate feedback into the development of Micro-Guardrail customization tools. This ongoing dialogue can help ensure that the platforms remain adaptable and relevant to diverse global requirements.
By adopting these principles, major AI LLM platforms can empower global businesses to leverage AI technologies in a way that aligns with their localized needs and values, while also upholding the principles of global diversity of thought and free speech. This approach recognizes that the definition of bias, appropriateness, and other ethical considerations can vary significantly across different contexts, and it is the responsibility of each business to define and implement guardrails that are suited to their unique environment.
Ethical Bias: A Necessary Consideration for Lawyers and AI Policy Makers
While the concept of bias is often viewed negatively in the context of AI, it is essential to recognize that ethical bias can be a necessary and valuable tool in AI implementation globally. For example,. “Ethical bias” refers to the intentional and transparent incorporation of specific viewpoints or perspectives into AI outputs to align with the goals of an organization. This type of bias is distinct from unintentional or harmful biases that can lead to discriminatory or unfair outcomes.
For example, companies using AI LLM APIs and Micro-Guardrails are well advised to avoid brand disparagement or in many instances disparaging other brands. Religious organizations are well within their rights to temper LLM powered output for meet their own compliance standards and to promote their own views. Lawyers have a professional duty to represent their clients' interests and present their cases in the most favorable light possible subject to the rules of ethics, like the avoidance of erroneous citations. In this context, the ability to incorporate ethical bias into AI systems, like plaintiff or defense oriented AI systems, can be crucial. By leveraging ethical bias Micro-Guardrails, lawyers can use LLMs to generate arguments, evidence, and narratives that effectively support their clients' positions.
In other words, one must think about the nuances of bias and acknowledge on some level that ethical bias will commonly be an important AI use case and ought to be implemented in a responsible manner.
Conclusion:
The Law of Guardrails represents a crucial step towards ensuring the ethical and compliant development of AI technologies. This new legal paradigm aims to mitigate the risks associated with AI applications, fostering innovation while upholding ethical and legal standards. As AI continues to evolve, the role of guardrails will become increasingly important in shaping the future of technology and society. Collaborative efforts among lawyers, policymakers, and technologists are essential to develop and implement effective guardrails that balance innovation with freedom of speech and responsibility, ensuring that AI serves the greater good. Lawyers and policy professional will play a crucial role in determining Ethical Bias and responsibly implementing the Law of Guardrails.